From Bedside to Bench: How Human Clinical Trials Can Inform Your In Vivo Studies
Developing new medicines is an expensive business and requires thousands of hours of work over many years. Despite this, the chance of a new drug being approved after entering clinical trials is only around 8%1. This low success rate can be attributed to the ‘translation gap’ - the gulf between the promise of a new therapy in preclinical models and how well it performs in patients. The translation gap not only increases costs - the average price of bringing a novel drug to market runs close to $1 billion2 - but also wastes time and, importantly, delays the availability of new drugs to patients.
New tools and perspectives are urgently needed to close the translation gap and ensure that our preclinical research is targeted and predictive. Here, we explore a ‘bedside-to-bench’ approach, which feeds information from the clinic back into preclinical experimental design and enables continuity from animal models to human outcomes.
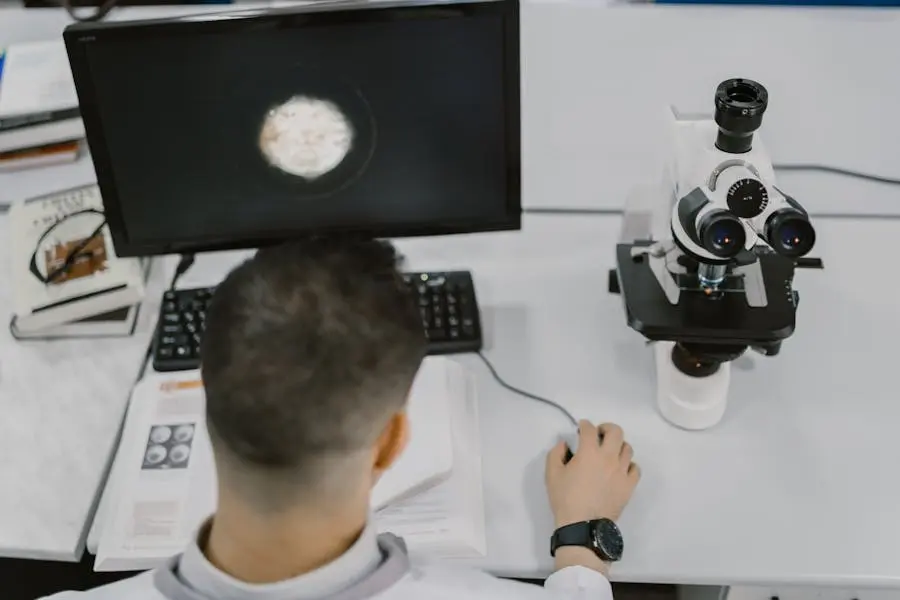
Shifting the Perspective on Translational Research
The ‘bench-to-bedside’ pathway describes the process by which findings in the laboratory, from cell-, animal-, or computer-based models, inform how we treat patients in the clinic: what biomarkers we look for, what drugs we use, and how we understand disease development. Typically, comprehensive data from preclinical tests, including animal models, is necessary for new therapies to enter clinical trials. However, assessing how well preclinical models can predict the efficacy of a treatment in the clinic is impossible if information only flows in one direction.
By reversing this traditional pathway, we can understand how well our preclinical models translate to the clinic: which models reliably predict the action of a drug in humans and which only serve to widen the translation gap.
Why Human Clinical Trial Insights Matter to Preclinical Researchers
While there are a variety of reasons why preclinical models can become widely used, often it is a system’s reproducibility or ease of use that leads to widespread adoption. Incorporating clinical data when assessing a model can help validate findings and challenge assumptions. For example, C57BL/6 (black 6) mice are the most widely used mouse strain in preclinical research3. Often, genetic uniformity is cited as a reason for their ubiquity, enabling highly reproducible experiments across labs. However, a lack of genetic diversity in models has been highlighted as a potential reason for failures in translating preclinical findings, for example, in Alzheimer’s research4. Taking this into account, researchers have sought to use a variety of models with a range of genetic backgrounds5.
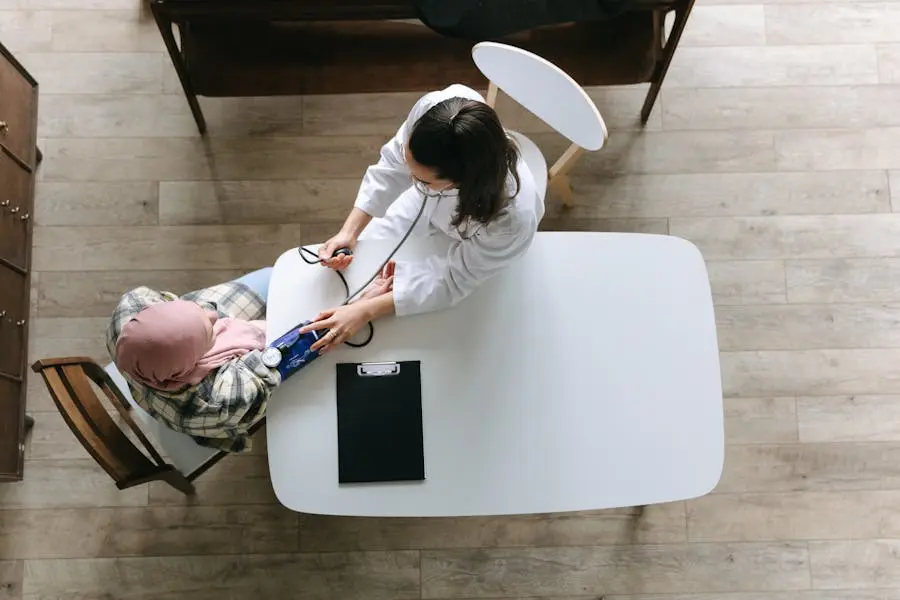
As such, understanding clinical outcomes can inform future animal model selection and experimental design, as well as helping to optimize and strengthen existing protocols6. This retrospective approach supports the refinement of existing models, encourages the development of more predictive systems, and ultimately fosters a more iterative, feedback-informed research cycle that better aligns with real-world outcomes. In doing so, we can shift from assumption-based design to evidence-based refinement.
Introducing ModernVivo’s Clinical Trial Citation Feature
With this in mind, ModernVivo has developed the new clinical citation badge, which indicates when an in vivo paper has been cited by a human clinical trial. When the badge appears next to an article, just one click will bring up clinical trial information and DOI. You can even filter your search to focus on in vivo studies with a clinical citation, helping you pinpoint research with real translational impact.
Get in touch with the ModernVivo team today and book a demo to see how you can incorporate a bedside-to-bench approach into your experimental design literature reviews.
AI Disclosure: Some of this content was generated with assistance from AI tools for copywriting.
References
1. Thomas D, Chancellor D, Micklus A, et al. Clinical development success rates and contributing factors 2011–2020. BIO Inf Pharma Intell QLS Advis. Published online 2021.
2. Sertkaya A, Beleche T, Jessup A, Sommers BD. Costs of Drug Development and Research and Development Intensity in the US, 2000-2018. JAMA Netw Open. 2024;7(6):e2415445. doi:10.1001/jamanetworkopen.2024.15445
3. Bryant CD. The blessings and curses of C57BL/6 substrains in mouse genetic studies. Ann N Y Acad Sci. 2011;1245:31-33. doi:10.1111/j.1749-6632.2011.06325.x
4. Enhancing face validity of mouse models of Alzheimer’s disease with natural genetic variation | PLOS Genetics. Accessed April 17, 2025. https://journals.plos.org/plosgenetics/article?id=10.1371/journal.pgen.1008155
5. Yokoyama M, Kobayashi H, Tatsumi L, Tomita T. Mouse Models of Alzheimer’s Disease. Front Mol Neurosci. 2022;15. doi:10.3389/fnmol.2022.912995
6. Optimizing drug discovery: An opportunity and application with reverse translational research. Health Sci Rev. 2023;6:100074. doi:10.1016/j.hsr.2022.100074
AI Disclosure: Some of this content was generated with assistance from AI tools for copywriting.